- Nov 27, 2024
- 9 min read
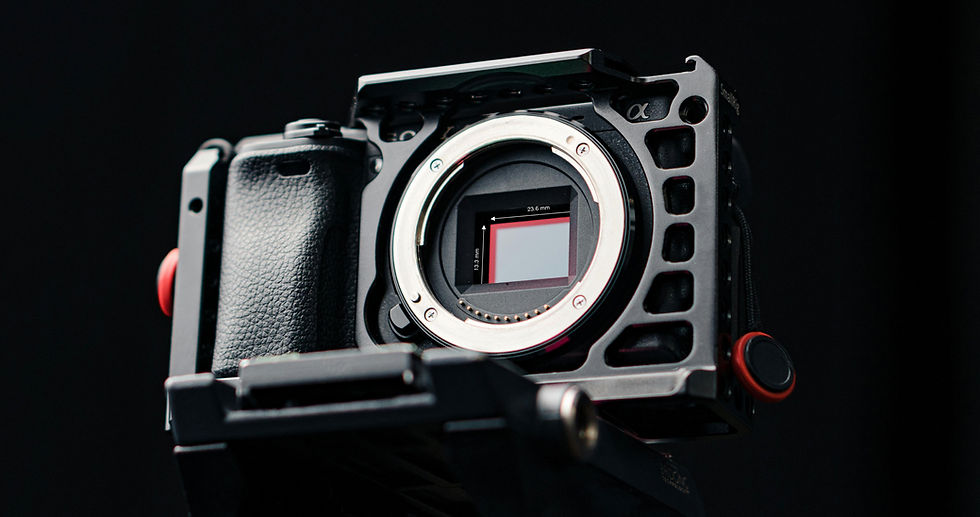
Navigate the learning article
Intro
What exactly is sensor size, and why does it matter for VFX, particularly when calculating the field of view for camera tracking?
This post explores the intricacies of sensor size. We’ll demystify key terminology, including "full-frame equivalent," "windowed," and "cropped" sensors. We'll also examine how metadata and other resources can help you out of a tight spot if you don’t have the info. By understanding and applying these concepts, you can gain greater control over your camera tracking projects.
What is Sensor Size?
So, perhaps a good place to start is defining what we mean by sensor size. Sensor size refers to the physical dimensions of a camera's imaging sensor—the part that turns incoming light into digital images. Usually, the height and width are measured in millimetres (mm); it is where the lens projects an image to be captured and converted into a digital signal. Sensor size affects how much of the lens's image is recorded, influencing the field of view, depth of field, and overall image quality.
How do I find out the size of a camera sensor?
While a quick Google search will provide the necessary information for most professional cine and mirrorless camera systems, it is worth delving deeper into the manufacturer's website to find the exact size. However, remember the considerations that will be discussed later in this article when finding out the sensor's 'actual size'. If a simple search doesn't yield results, the following links lead to excellent websites that cover the essential details you may need.
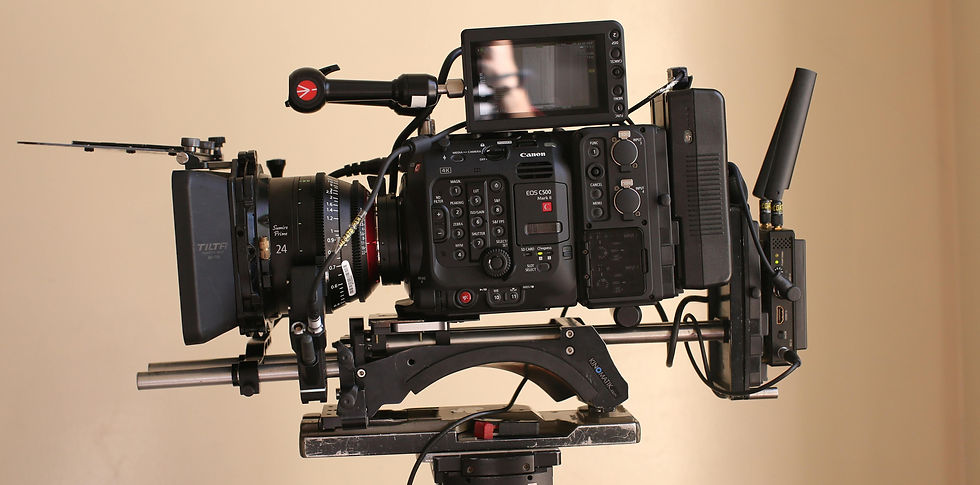
VFX camera database
The VFX Camera Database is a valuable resource that offers an extensive, mostly up-to-date collection of professional and prosumer cameras. What sets this site apart is its inclusion of detailed measurements, not only for the full active sensor area but also for windowed sizes in different recording modes.
DXOMARK
This website is a database primarily focused on testing camera sensor performance. However, under the specifications tab, it also provides key details like the actual sensor size and other useful data for matchmoving, such as rolling shutter performance. This resource is especially helpful for tracking phone footage, as it includes information on most of the latest phone cameras, including sensor sizes and field-of-view equivalence.
CINED
CINED is a production-focused website offering in-depth testing and reviews of the latest camera gear. While it primarily focuses on sensor performance, much like DXOMARK, it also provides valuable details on sensor size and windowing for various popular camera systems, including some phones and mirrorless cameras—making it a useful resource for camera tracking tasks.
Why is ‘Sensor Size’ important for camera tracking?
Camera tracking applications like PFTrack require the camera's field of view (FoV) to accurately track and solve a shot. The FoV is determined by both the lens's focal length and the sensor size. However, precise knowledge of the sensor size remains crucial for ensuring the virtual camera accurately replicates the real-world camera's perspective and movement. Without this, discrepancies in scale, position, and motion can misalign digital assets, disrupting the realism of the final shot.
Sensor Size Considerations
Loose terminology for the sizing of an imaging sensor
You’ve probably come across terms like Super35, Full Frame, and large format to describe the size of an imaging sensor in cine or still cameras. While it might seem straightforward to search "What is the size of a Super35 sensor?" and rely on the results, the information can often be inconsistent due to manufacturers' generalised and imprecise terminology.
To illustrate, suppose we have a camera with a 24mm focal length, and the camera in question is a Sony PMW-F3, which uses a "Super35" sensor. If we rely solely on Google's definition of Super35 based on the traditional film format, we might calculate the following horizontal and vertical field of view:
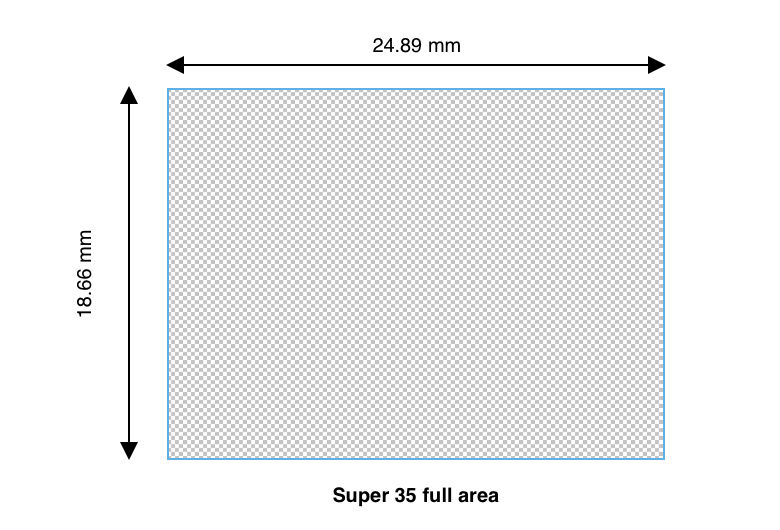
Super35 format size: 24.89 mm x 18.66 mm
Horizontal FoV: 54.82°
Vertical FoV: 42.49
Delving deeper into the manufacturer's sensor specifications reveals that its size is not identical to a true Super35mm sensor; instead, it has been rounded up and features a different aspect ratio. This discrepancy impacts calculations, resulting in a field of view that is 2.46° narrower horizontally and 11.52° narrower vertically than anticipated.
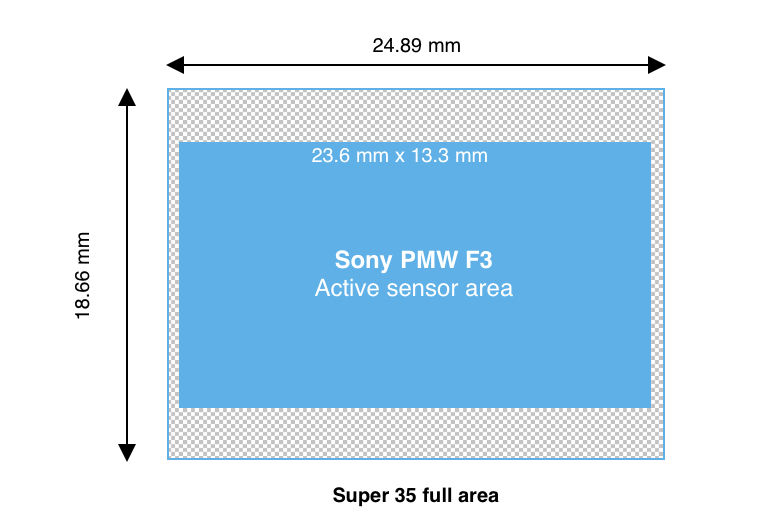
Actual sensor size: 23.6 mm x 13.3 mm
Horizontal FoV: 52.36°
Vertical FoV: 30.97°
While this may not seem significant, even small sensor size discrepancies can impact your solve's overall accuracy. This is especially true for smaller sensors, such as those used in drones and cameras built into phones, where precise field of view (FoV) calculations are critical.
The term "Large Format" adds further confusion, as it has come to refer to any sensor larger than 36x24mm without clearly defined upper limits, complicating efforts to strictly define sensor size for camera tracking. The situation becomes even more complex when considering sensor crop and various windowed shoot modes.
Windowed, Scaled, and Crop Modes: Effects on FoV
If you have looked up the size of the image sensor in the camera that shot your clip and entered the information, and things just don’t seem to be making sense or working, it might be because your camera is shooting in a mode that affects the FoV of your image.
Full Area Vs Active Area
The difference between the active imaging area and the full sensor area lies in how much of the sensor's surface is actually used for capturing an image versus the total physical size of the sensor itself.
Full Sensor Area: This refers to the total physical dimensions of the sensor, including all of its pixels and regions, whether they are used for capturing an image or not. The full sensor area accounts for every part of the sensor's surface, including pixels reserved for other functions (such as calibration or stabilisation) or areas that may be masked out during image capture.
Active Imaging Area: This is the portion of the sensor that is actively used to capture an image. It defines the region where incoming light is collected and converted into a digital image. Due to manufacturer-specific design choices, cropping, or masking, the active imaging area can be smaller than the full sensor area. This distinction is important in applications like camera tracking, as it directly affects how the image is projected onto the sensor and impacts field-of-view calculations. When entering information about the sensor in your camera, it is always important to use the ‘Active Imaging Area’ over the ‘Full Sensor Area’ where possible for best accuracy.
Windowed Sensor Mode
Sensor windowing occurs when only a portion of the imaging sensor is used to capture an image, effectively "cropping" the sensor's active area. This is common in cine cameras when recording RAW and selecting a resolution or format other than the sensor's native resolution. Instead of resampling the full sensor, the camera activates a smaller portion of it, which alters the field of view. Similarly, sensor windowing is often used to achieve very high frame rates, as processing data from a smaller sensor area reduces the hardware's workload.
For instance, the RED MONSTRO 8K sensor, measuring 40.96 x 21.6 mm, utilises its full area when shooting at its maximum resolution of 8K (8192 x 4320). However, the camera applies sensor windowing to achieve lower resolutions, using only a portion of the sensor’s area.

RED MONSTRO Windowed shooting modes:
6K shooting mode (6144 x 3240), area is 30.72 x 16.20 mm
5K shooting mode (5120 x 2700), area is 25.6 x 13.5 mm
4K shooting mode (4096 x 2160), area is 20.48 x 10.80 mm
Sensor windowing may also be necessary when using lenses for smaller imaging circles, such as a Super35 (31.1mm) optic on large format sensors).
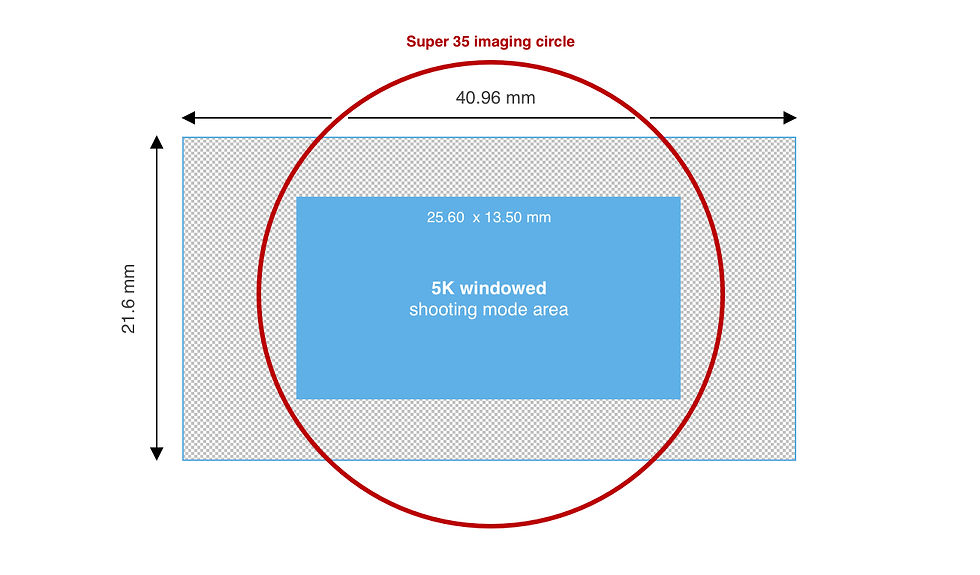
Fortunately, PFTrack allows you to input the full area of a sensor and account for any windowing that may be happening with your camera using a separate input for the windowed area.

Scaled Sensor Mode
Sensor scaling is a simpler concept compared to sensor windowing. It involves resampling the image captured by the entire sensor area to a lower resolution while preserving the full active sensor area on one or more axes.
Full Area Resampling
Full-area resampling takes the image captured by the entire sensor and downsamples it to a lower resolution without altering the active area or the FoV. For example, a sensor with a native resolution of 3840x2160 might be resampled to 1920x1080, maintaining the full sensor area while reducing the pixel count.
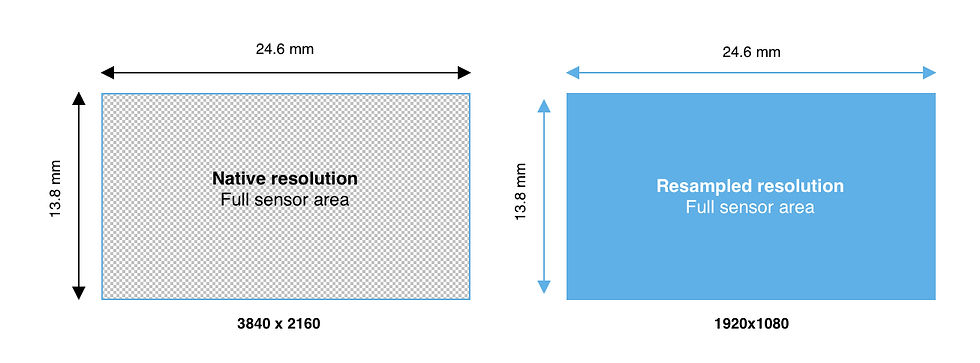
Aspect
Sensor scaling can also account for changes in aspect ratio. For instance, a sensor with a native aspect ratio of 1.78:1 (16:9) may crop or scale the image at the top and bottom to produce a 1.85:1 aspect ratio while maintaining the full sensor width and horizontal FoV. You can enter the sensor's width, and PFTrack will calculate the height automatically. Changing aspect scale can also happen vertically; for example, if you have a native 1.85:1 sensor, the scaling may crop the sides to reach a 1.78:1 aspect ratio (see anamorphic).

Anamorphic
Anamorphic scaling preserves the full sensor height and vertical FoV, while the sides are scaled/cropped to achieve the desired anamorphic recording ratio, such as 1.33:1. You can enter the sensor's height, and PFTrack will calculate the width automatically.

It's important to note that resampling a 3840x2160 resolution sensor using the full sensor area to 1920x1080 is not the same as using a 1920x1080 windowed shoot mode, where only a portion of the sensor's full area is utilised. The two methods will result in very different fields of view (FoV).
Metadata
A key advantage of using an application like PFTrack is its ability to read metadata from formats like DPX and EXR and many camera RAW files. But why is this important for determining a camera's sensor size? Metadata often contains critical details, including the camera model and shooting mode, which can help quickly and accurately identify the sensor size from the data or use it to select an appropriate sensor preset.

A multiformat sensor?
Don’t worry this sounds more complicated than it is. The term refers to using a slightly larger sensor than standard, allowing the camera to “window” the sensor to achieve various aspect ratios, formats, and frame rates directly in-camera rather than capturing the full sensor area and cropping it later.

A prime example is the ARRI Alexa 35, which utilises its full active sensor area of 27.99mm x 19.22mm in Open Gate mode and dynamically windows/scales the sensor to accommodate common standards at the correct measurements.

For instance, the 1.78:1 mode uses a 24.88mm x 14.00mm area, while the anamorphic 6:5 mode employs a 20.22mm x 16.95mm area. This adaptability ensures the sensor can handle diverse applications, leveraging its entire surface or specific regions to deliver the desired field of view and resolution for each format using the correct imaging circle.
Full-frame equivalent or the actual sensor size?
If you've searched everywhere but can’t find information about your sensor size, you might still have a "full-frame equivalent" focal length to work with.
The term "full-frame equivalent" refers to the focal length of a lens on a camera with a sensor size other than full-frame (36mm x 24mm) that produces a similar field of view to a lens on a full-frame camera. Essentially, it allows for comparing how a lens on a smaller sensor camera would behave if mounted on a full-frame camera. Manufacturers often use full-frame equivalence to simplify marketing, particularly in systems with integrated optics, such as handheld gimbals and drones. However, this approach can obscure the true sensor size.
So, can you rely on full-frame equivalence instead? The answer is both yes and no. For example, the DJI Osmo Pocket 3 does not readily disclose its sensor size, but it states that the combination of its sensor and optics produces a full-frame equivalent field of view to a 20mm lens. Using this information, you could input the horizontal size of a full-frame sensor (36mm) and a 20mm focal length to estimate the field of view. However, this assumes the 20mm equivalence is precise. Manufacturers often round up or down to the nearest common photographic focal length for simplicity. While such minor differences are negligible for everyday filming or photography, they can lead to inaccuracies in precision workflows like camera tracking.
Full-frame equivalence can provide a starting point if no other data is available. However, be cautious, as it might not deliver the accuracy required for tasks like camera tracking.
Wrap Up
In conclusion, we hope this post has clarified some of the challenges in identifying the correct sensor size for your camera while providing a foundation in key concepts and terminology.
Whether working with high-end cine cameras or smaller devices like drones, understanding and applying sensor size information is essential for accurate tracking. Leverage resources like the VFX Camera Database and DXOMARK to quickly access precise sensor specifications for your projects.
Finally, remember that PFTrack offers powerful tools for calibrating your camera body, and the Auto camera model can be a reliable fallback when all else fails. Armed with this knowledge and the right tools, you'll be well-equipped to tackle your next camera tracking challenge with confidence.
Links
To explore PFTrack and the topics discussed in this article, you can download it using the links below and start exploring in discovery mode for free.
Download PFTrack now