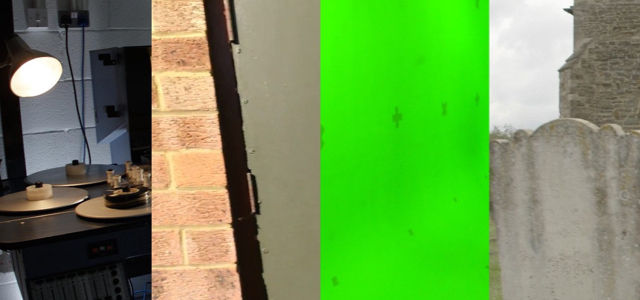
Matchmoving is becoming more and more of an automated process of tracking and solving. But there are still cases where the keen eye of the matchmove artist can save time by spotting potential issues that could derail your tracks and solve them. This post will list what to look for to identify which clips need your attention.
1 — Lens distortion
Lens distortion is an optical aberration that causes straight lines to appear curved in photos or films, and it is easy to see how this can cause issues for the matchmove artist.
Trackers along a straight line in the real world are no longer on a straight line in the resulting distorted image, and the effect on a camera track can, at best, produce false positives or, in the worst case, cause the 2D tracking to fail altogether.
Lens distortion can be recognised by looking for straight objects at the edges of the frame, such as the beam in the image below.
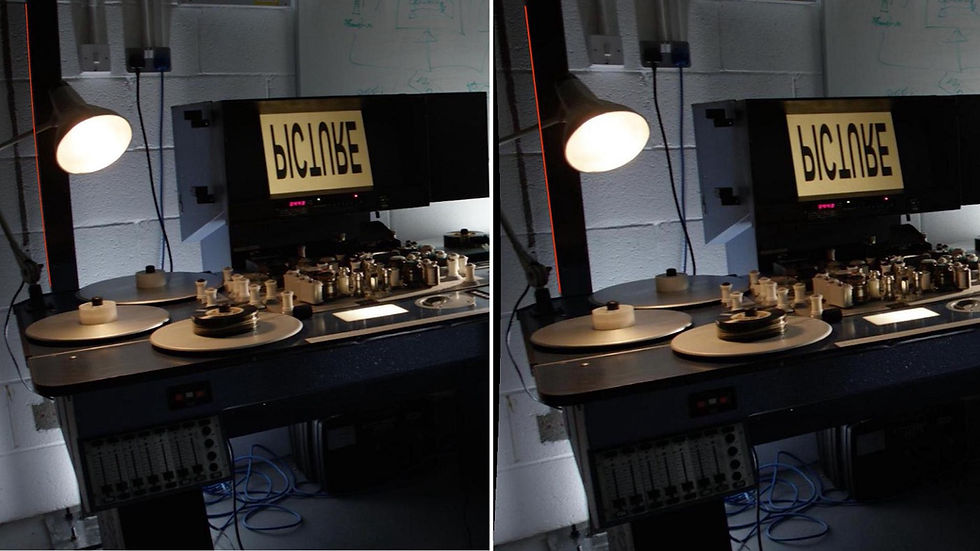
Due to the 3D representation of trackers that should be in a straight line now being on an arc and not truly reflecting the real-world scene, the camera solve will fail when it becomes impossible to line the virtual camera up with the distorted tracking points.
Fix?
Film and television audiences are used to a certain amount of lens distortion in their viewing experience, and any CG must be distorted in the same way as the background plate to blend in perfectly. The trick is to undistort the image plate BEFORE carrying out any tracking/matchmove operations, then use the calculated distortion models further into the VFX pipeline.
All good matchmoving software has distortion pipeline tools built in, which allow the distortion of the background plate prior to tracking and the ability to pass the distortion metrics (more commonly supported through ST Maps) further into the VFX pipeline, usually the composting software.
2 — Rolling shutter
Like lens distortion, rolling shutter results from limitations of the image capture technology employed to shoot the footage. The effect of rolling shutter occurs when different lines of the image sensor are recorded at slightly different times, which commonly happens with CMOS sensors.
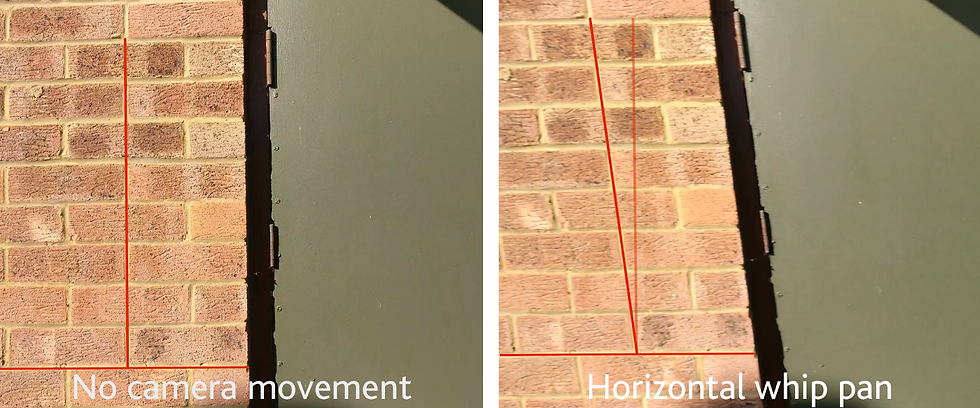
The effects of shutter roll are most noticeable with whip-pans or rapid translations. If the camera sensor records the image line by line during such fast movements, different parts of a frame are recorded at different times and from different camera locations.
Unfortunately, a bad rolling shutter can render your footage almost unusable for motion effects such as tracking and titling, not just because the distorted image will cause tracking to fail but also because it is virtually impossible to match any form of CG to the unpredictable distortion.
Fix
The best fix is to sidestep any capture technology that produces this particular effect and opt for a better-quality device. However, the fix-it-in-post mentality that can sometimes occur means the VFX departments get what they are given. Fortunately, there are fixes out there.
To make a usable image, you must reverse-engineer a unique camera position for a single frame when no such position exists. Shutter roll must be treated before the tracking, so matchmoving applications can rely on all scanlines of a single frame to represent the same time and location.
Shutter roll became such a big issue that numerous plugins from third-party vendors are available to provide fixes, with varying results. PFTrack has a tool built in to undistort the background, which can be passed down the tracking tree, and other matchmove apps can deal with footage similarly.
After undistorting the rolling shutter and tracking, you will need to provide the resulting undistorted background plate further into the VFX pipeline for any compositing, etc. to be carried out. Unlike lens distortion, it is not usual to re-introduce the distortion characteristics.
PFTrack’s Shutter Fix node can be used to reduce the effects of rolling shutter.
additional rolling shutter ref — https://en.wikipedia.org/wiki/Rolling_shutter
3 — Lack of features
Matchmoving applications rely on tracking static object features within the image. From the way these features move through an image sequence, the matchmoving application reverse engineers how the camera was moved to film it and even some properties of the camera itself, such as focal length. Ideally, the features to be tracked will be well distributed over the entire 2D image, as well as the 3D space of the scene.
So, the key to a successful auto-track and camera-solve is to have plenty of well-spread, trackable features in your clip. A trackable feature can be virtually anything that stands out in the image, such as the corner of a window.
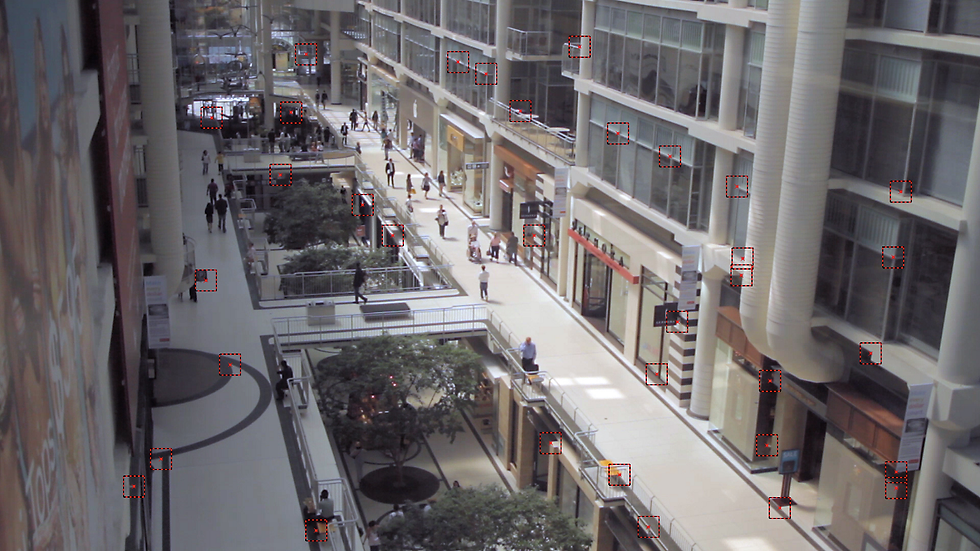
No background detail
Uniform backgrounds, such as a green screen used in many VFX shots, however, don’t have as many features as in the example above. There is nothing to track in the worst cases, such as in the clip below. This clip will require some manual work to get a working camera.
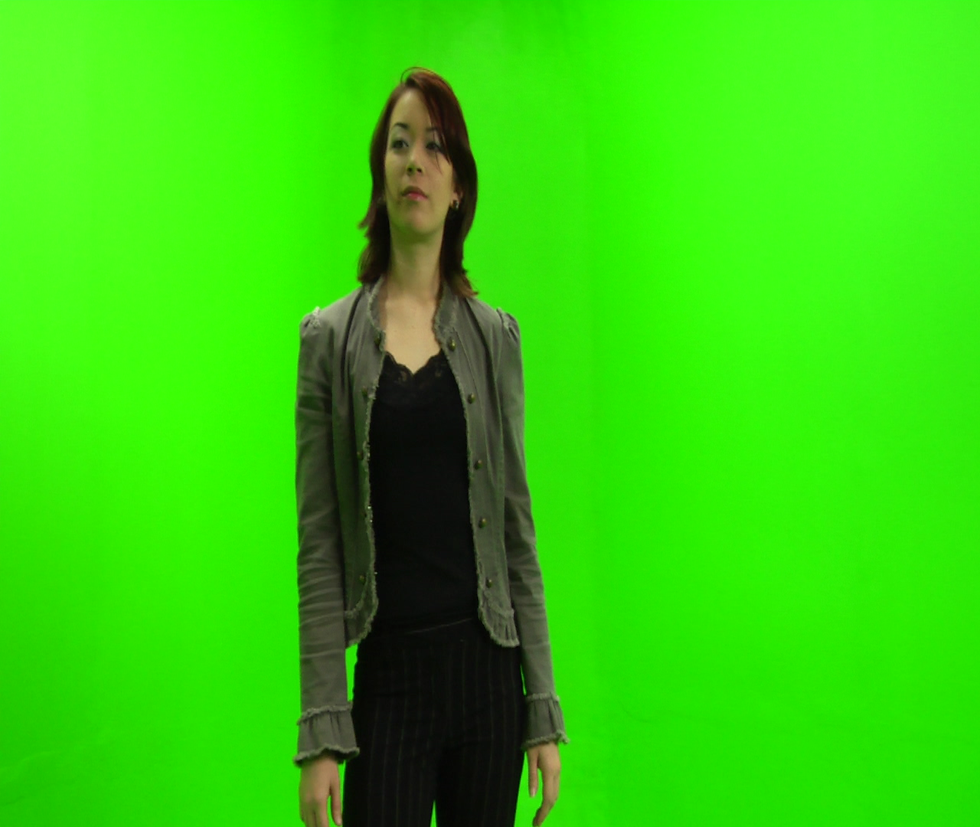
On the other hand, even green screens do have tracking markers in many cases, but due to the nature of green screens, these markers will not always sufficiently stand out.
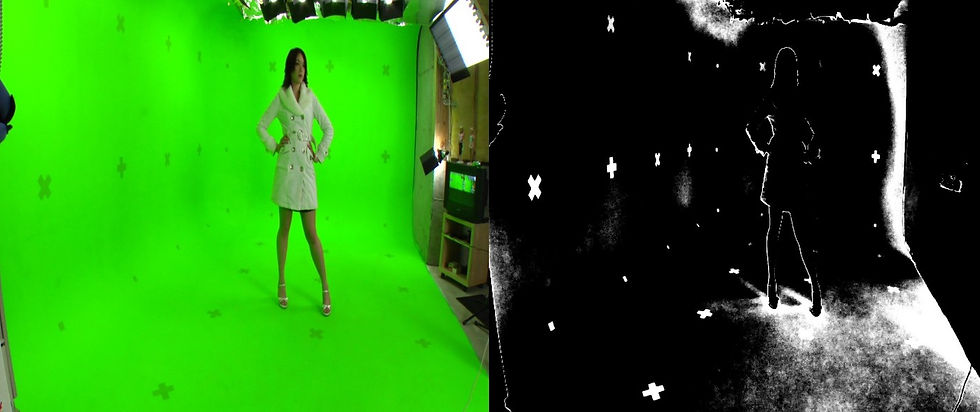
Fix
The clip can be altered in many cases to make it more visible, as in the example above.
Motion blur
Another common case that can result in a lack of trackable features is motion blur caused by a fast-moving camera. As such, motion blur makes it harder for an algorithm to locate trackable features. Any features that may be found are also harder to track due to the fast camera motion.
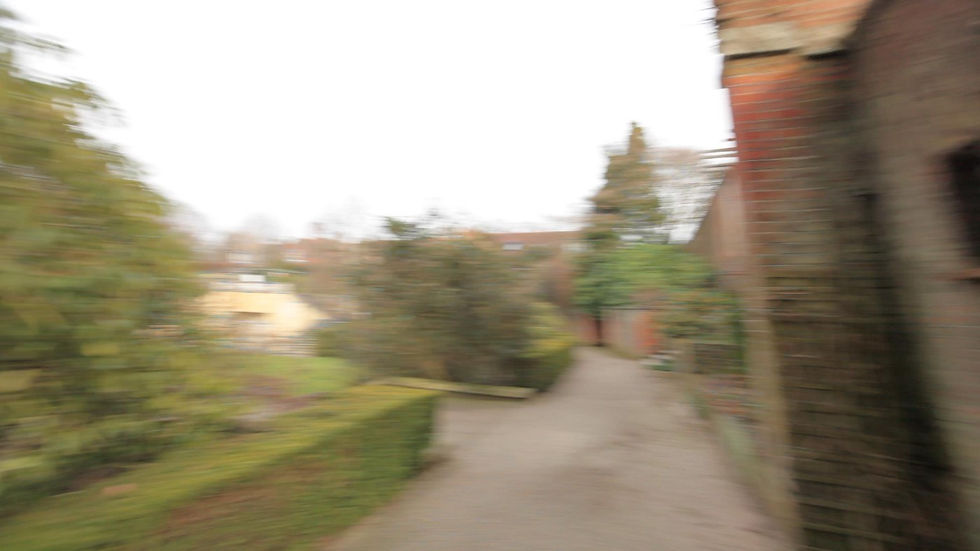
Fix
You may be able to recover enough detail for a track through image processing, but in many cases, clips with heavy motion blur will require manual trackers to get the best result.
4 — Incorrect features
In some cases, there may be plenty of features to track, yet these features would not feed the correct information to the matchmoving applications. To be of any use to solve for a camera, trackers must represent the same real-world 3D position throughout the clip. Below are some examples of where this is not the case.
Too much movement
One obvious example of trackers not sticking at what represents the same real-world position is when there is movement inside the shot, such as moving cars or people. In an exterior scene, these could also be branches of trees subtly swaying in the wind. Even though they may appear not to move very much, they can pose a problem if too many trackers are on them.
The clip below shows an example of a moving person. While these trackers cannot be used to solve a camera, they would still be helpful to solve the object’s motion in a later step.
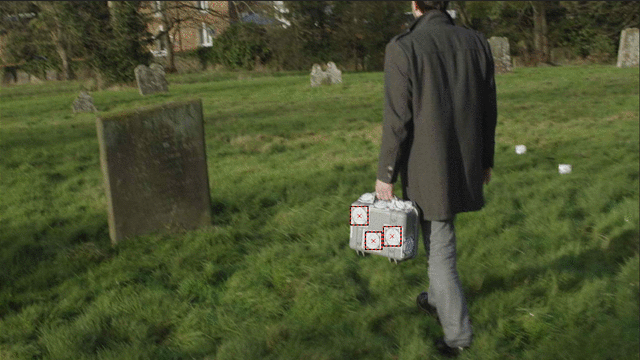
Fix
If a shot contains too much motion, the moving objects may have to be masked out before tracking or any trackers on such objects removed before feeding them into the camera solver. In many cases, however, the consistency parameter in PFTrack’s Auto Track node can automatically eliminate independently moving trackers.
False corners
Another example where trackers do not provide helpful information, neither for camera nor object tracks, is false corners. False corners occur when two objects at different distances from the camera overlap. Tracking algorithms could interpret the intersection of these two objects as a trackable feature. Solving algorithms, however, expect features to represent the same 3D real-world position, which is not the case for false corners.
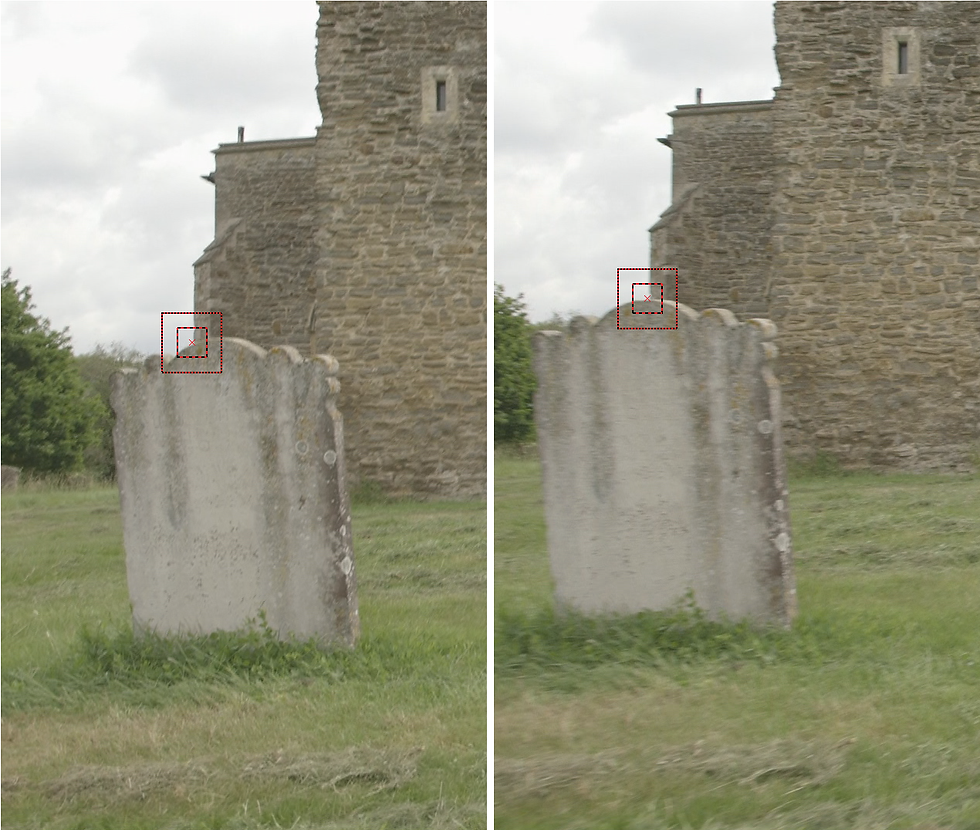
Fix
This issue requires an observant operator to spot suspicious trackers. Turning on tracker motion prediction in PFTrack’s Auto Track or Auto Match node may help avoid tracking false corners, as can the Auto Track node’s consistency setting.
5 — No Parallax
Matchmoving relies heavily on parallax, the familiar effect that objects far away move more slowly than objects closer to us. For camera tracking, the application uses this knowledge to estimate the relative distance of trackers from the camera and determine how the camera moves. But there are types of shots that do not exhibit any parallax.
Locked off shots
Without any camera motion, background features will not move at all, which means features further away cannot move slower than features closer to the camera.
Zoom shots
At first glance, it may look like motion, but zooming into a locked-off camera does not exhibit parallax. Zooming only magnifies a part of the image, and all objects inside that part keep their relative positions. The following example shows the different results you get from a zoom shot compared to a dolly shot, where the camera moves forward. Note how in the dolly shot, the objects move relative to each other, and, as a result, more of the circled object is revealed at the end of the shot.

Nodal pan
Nodal pans are a third example of shots that don’t contain parallax. The easiest way to imagine a nodal pan is a camera mounted on a tripod with no horizontal movement. This rotational motion of the camera does not create any parallax, as illustrated in the clip below.
While most tripod shots are not actual nodal pans, they must rotate the camera around its optical centre. They often still do not contain enough parallax to solve for accurate 3D tracker positions.
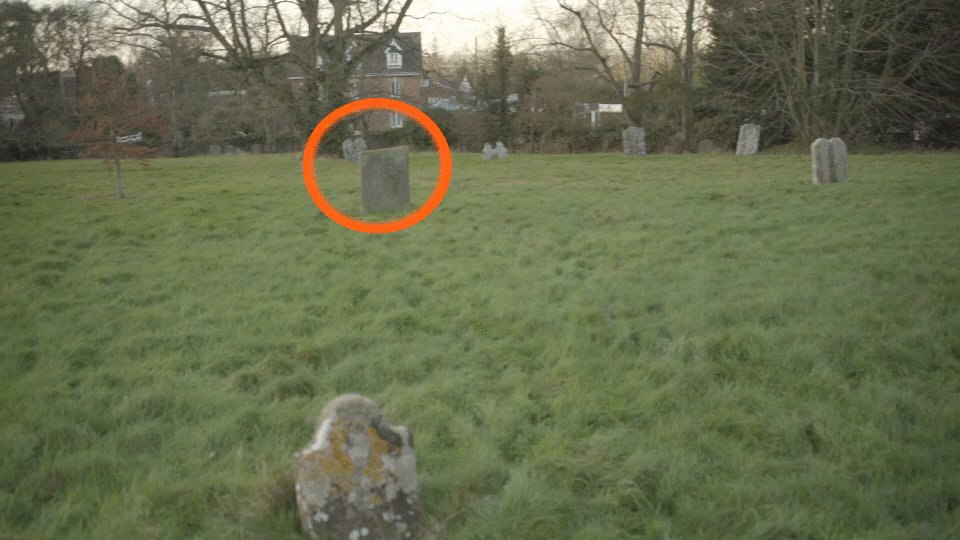
Fix
Introducing additional views of the scene, such as still images shot from a different position, will let you extract 3D data from nodal pans.
Conclusion
Spotting these issues early can help you distinguish easy-to-track shots from those that need extra care in matchmoving. The Pixel Farm’s matchmoving application, PFTrack, provides tools to help you mitigate these issues (as outlined in the fix suggestions) and solve many difficult situations.
Start now and download PFTrack today to explore its powerful features for free in discovery mode!